Artificial Intelligence for Insurers: Understanding the Ethics of Use
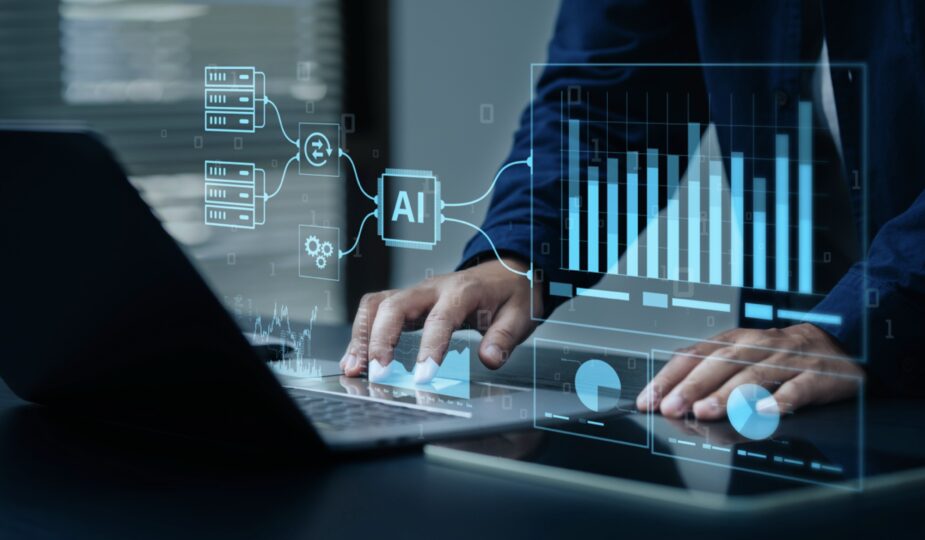
SCOR’s AI and the Future of Insurance publication discusses several critical points to consider in adopting artificial intelligence (AI) to insurance businesses. In this article, we’ll examine SCOR’s insights into the ethical aspects insurers must keep in mind in the AI era.
Any new technology adoption comes with initial fears or concerns. In adapting AI models to business, concerns specifically point to AI and ethics. Some fear that AI could introduce bias, discrimination, and unfair insurance practices.
To address these concerns, insurers must reevaluate their pricing and risk selection practices for fairer insurance when they develop their AI deployment strategy. But how do we do it? No one knows the answers yet.
Fairness in insurance risk selection and pricing is not a new topic in the highly regulated insurance industry, but the recent emergence of AI is bringing additional pressure. Insurance is a data-driven industry; hence, processes are already in place to monitor and control model accuracy and fairness.
However, existing techniques need to be strengthened as the powerful AI model is capable of learning discrimination patterns from the input data, even if protected fields are not present. For example, in auto insurance, an AI model can guess or proxy the gender of the applicant by looking at the policyholder’s car features.
Read: AI and the Future of Insurance
Recent AI buzz has raised awareness of potential biases originating from data used by algorithms. We need to remind ourselves that AI models are not intentionally discriminating against anyone or anything. They are simply reflecting the data they have been trained on.
For example, imagine building a company profile: if the majority of the company’s employees are white males, a model trained to generate a typical profile of its employee will show a picture of a white man unless it is given specific de-biasing instructions. For decades, actuaries and data scientists have been tackling the issue of fairness by removing sensitive variables from the dataset, which could lead to unfair prices.
However, with the current rapid expansion in AI capabilities, there is an urgent need to find a new technique to build a better fair pricing model. To see how much sensitive variables such as gender, race, origin, or marital status could affect fairness in insurance modelling, a team of researchers, including SCOR’s data scientists, conducted a model simulation study.
The study found that simply removing sensitive variables does not necessarily lead to a fairer model, as they are often correlated with other risk factors. To experiment with possible solutions, the team developed a new algorithm to mitigate proxy discrimination in its risk modelling.
Figure 1 shows the real-case application for a fairer risk selection of patients with non-metastatic melanoma of the skin. As shown in the boxed areas of the two similar charts, the variable transformation has successfully annulled the correlations between the non-sensitive and sensitive variables. This first solution development for using linear models set some first principles in the preparation of the data before modelling.
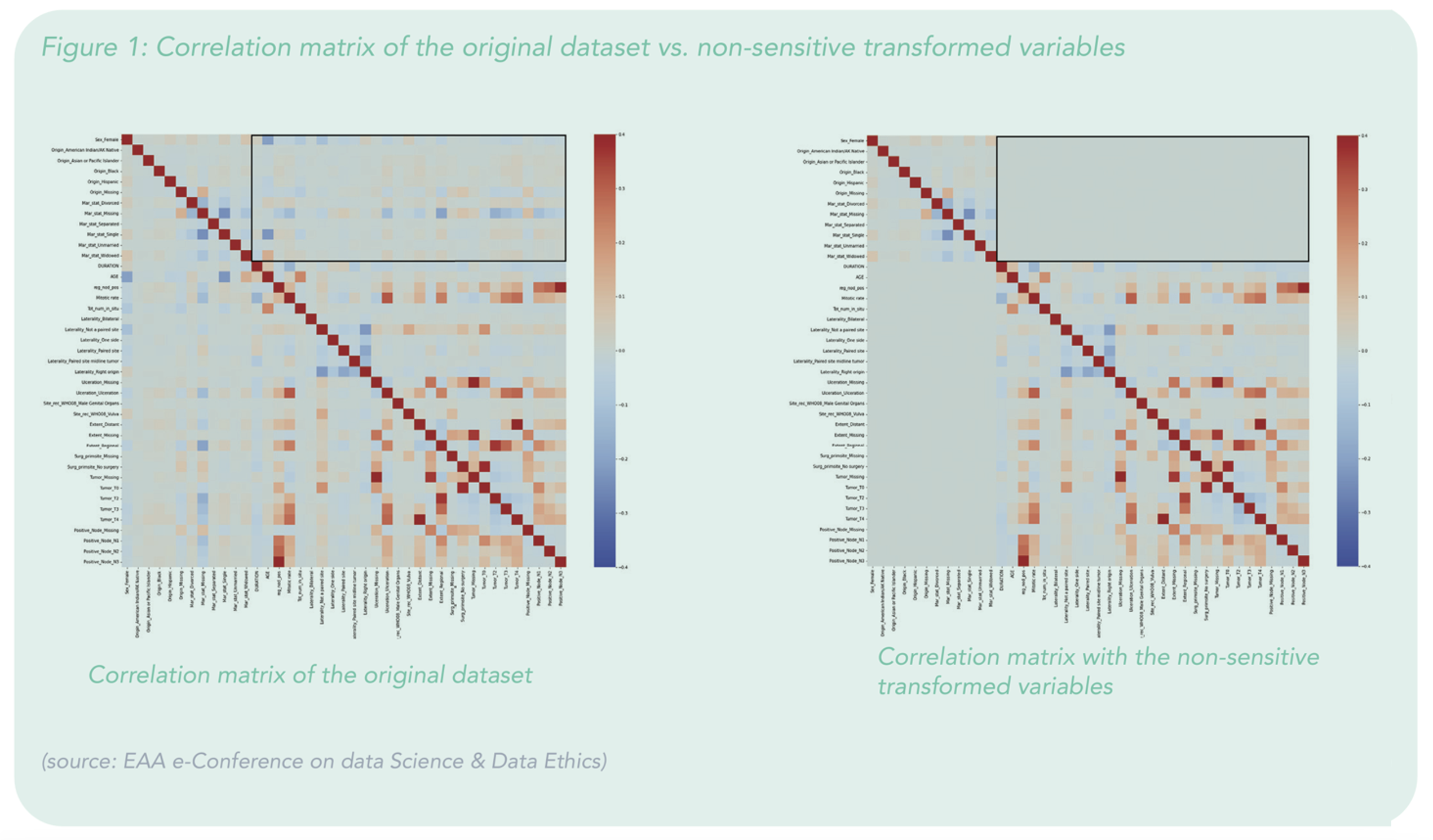
In coming years, it is inevitable that more extensive usage of AI will expose the insurance industry to discover bias in their data. We will need to be more vigilant to avoid proxy discrimination and maintain fairness in our decision-making process. It is highly difficult to find a globally unified definition of fairness in insurance pricing and risk selection, as it greatly differs by country and is not easy to define or unanimously agree on. The definition is influenced by market-specific factors such as regulation, social structure, consumer sentiment, scientific advancement, technical capabilities, etc.
We can test AI models using different measures of fairness, but they could still lead to contradictory conclusions. Even when we have a clear idea of what fairness might mean for a particular AI model, there remains a challenge in measuring it if the data is insufficient (e.g. no access to protected variables during model calibration) or contains biases. Ultimately, it is up to authorities in each market to determine the most appropriate definition and, therefore, which is the most appropriate and ethical modelling.
Read more about the opportunities, challenges, adoptions, and ethics of AI in SCOR’s AI and the Future of Insurance publication. To find out more about how our digital solutions can help you to support your policyholders and grow your business, head here.